
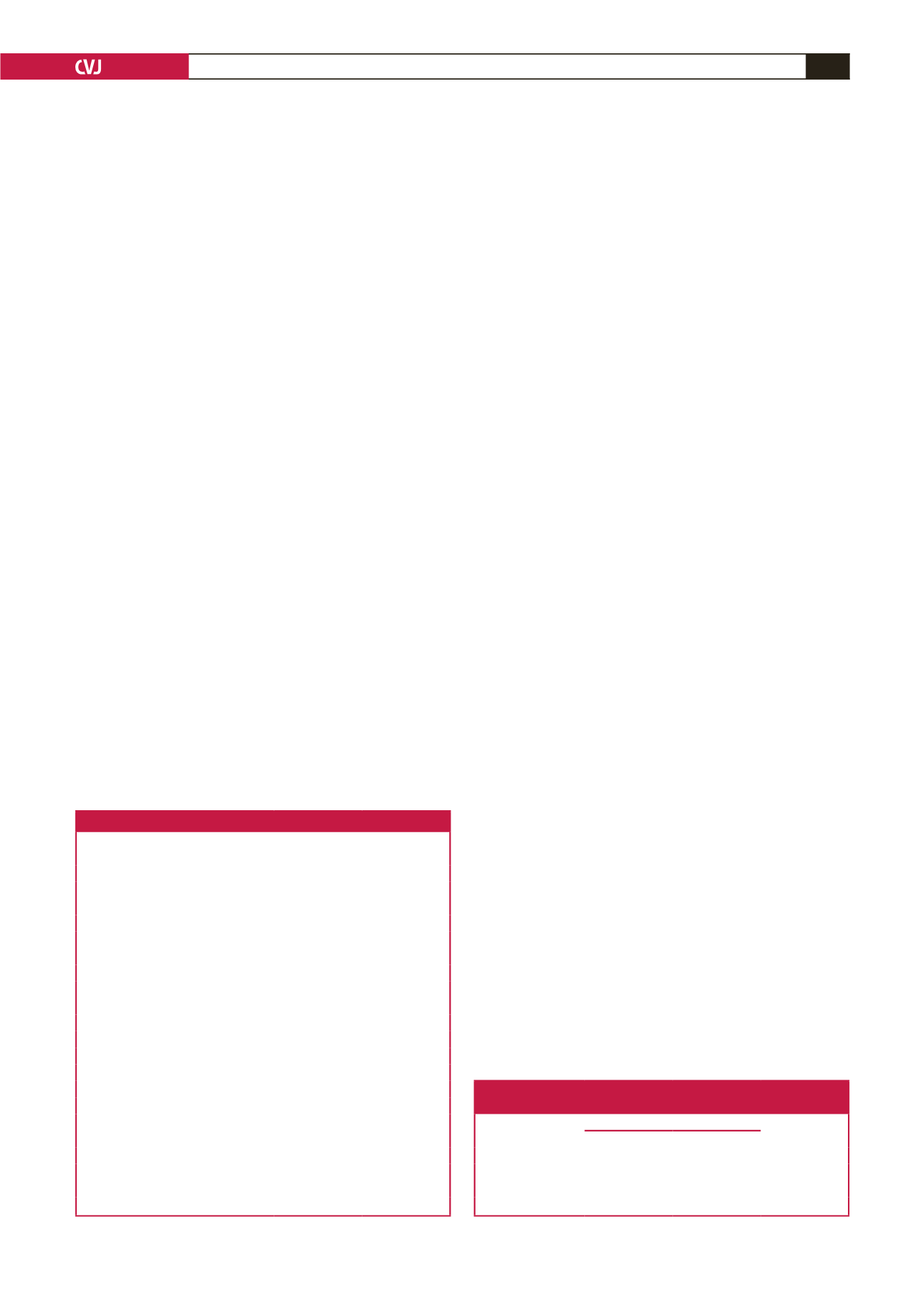
CARDIOVASCULAR JOURNAL OF AFRICA • Volume 30, No 3, May/June 2019
AFRICA
147
a value between the 85th and 95th percentile for age and gender
was considered overweight. Underweight was defined as a BMI
value less than the 5th percentile.
All subjects were classified as overweight and obese according
to the Cole
et al
. cut-off point.
19
The international cut-off points
for undernutrition (grade one, two and three) by gender for exact
ages defined to pass through BMI of 16, 17 and 18 kg/m
2
were
used.
20,21
Systolic and diastolic blood pressure (BP) were measured to
the nearest 1 mmHg by trained fieldworkers on all participants in
a seated position, with a calibrated M5-I digital automatic blood
pressure monitor. Three consecutive measurements were taken
and the mean of the last two systolic (SBP) and diastolic blood
pressure (DBP) values was calculated and used to determine the
blood pressure status. High blood pressure was defined as a SBP
and/or DBP value greater than the 95th percentile at the standard
age–gender–height percentile-specific blood pressure tables.
22
Statistical analysis
The chi-squared automatic interaction detection (CHAID)
decision tree analysis is a classification method for building
decision trees, using the chi-squared test to determine the best
next split. It first examines the cross-tabulations between each
responder and predictor variables, and chooses the predictor
variable with the smallest adjusted
p
-value. The CHAID decision
tree analysis was applied to identify factors and determine their
relationships with underweight children. In CHAID analysis,
underweight was the target variable and risk factors were
explanatory variables. SPSS version 25.0 (IBM SPSS Statistics)
was used for descriptive and CHAID decision tree analyses.
Results
Table 1 shows the general characteristics of the study participants,
including underweight status, gender, blood pressure status,
nutrition, school level and age group. Overall, 19.5% of the
children were not underweight, while 80.5% were underweight.
Hypertension was found in 1.3% of these children and 98.7%
were not hypertensive. Table 2 demonstrates the distribution of
hypertension status and childhood underweight. Although it
was not found to be significant, the prevalence of hypertension
was seen to be higher among underweight children (65.2%) than
those not underweight.
Results of the CHAID decision tree analysis are presented in
Fig. 1. The model demonstrated multilevel interaction among
risk factors through stepwise pathways to detect childhood
underweight. The model included gender, age group, school
level, and blood pressure and nutritional status. Before and after
adjusting for known confounders, predicting variable blood
pressure status was not found to be statistically associated with
childhood underweight. The final model showed that there were
four main predicting variables affecting childhood underweight:
the first variable was split on nutrition, followed by age group,
gender and school level, with a significance level of
p
<
0.05.
Looking at the underweight children who had moderate or
severe undernutrition, the model was able to predict that those
children would be underweight roughly 99.8% of the time. This
prediction applied to 509 children and the model was accurate
508 times.
Our results showed that in underweight children who had
normal nutrition and were between the ages of five and seven
years, we were able to accurately predict that they would be
underweight around 99% of the time. Alternatively, if children
were between eight and 10 years of age and still at pre-school, we
predicted that they would be underweight 88% of the time. This
applied to 146 children and we were accurate about 128 times.
Looking at underweight children who hadmild undernutrition
and were between the ages of five and 10 years, we were able to
accurately predict that they would be underweight 100% of the
time. Alternatively, if children were between 11 and 16 years of
age and were male, we predicted that they would be underweight
93% of the time. This applied to 219 children and we were
accurate about 204 times.
Discussion
Our results show a high prevalence of childhood underweight,
confirming previous findings,
23
and the continued existence of
underweight, among other health issues in young black African
populations.
24-26
The overall prevalence of childhood underweight
in our study was 80.5%. The high underweight prevalence in this
rural South African population may be indicative of lower socio-
economic status occurring in the context of low wealth index
and low intake of n-6 poly-unsaturated fatty acids as well as an
overall high rurality index, which has previously been associated
with underweight.
23,27
Table 1. General characteristics of the study participants
Variables
Number
Percentage
Gender
Male
934
51.6
Female
877
48.4
Age categories
5–7 years
140
7.7
8–10 years
649
35.8
11–13 years
989
54.6
14–16 years
33
1.8
Educational level
Primary school
1334
73.7
Pre-school
477
26.3
Underweight
No
354
19.5
Yes
1457
80.5
Hypertension
No
1788
98.7
Yes
23
1.3
Nutrition
Normal
671
37.1
Severe undernutrition
186
10.3
Moderate undernutrition
323
17.8
Mild undernutrition
631
34.8
Table 2. Chi-squared test for the association between
hypertension and underweight
Variables
Hypertension,
n
(%)
Chi-squared test
No
Yes
p
-value
Underweight
No
346 (19.4)
8 (34.8)
0.064
Yes
1442 (80.6)
15 (65.2)
Total
1788
23